18 Months Until Autonomous AI

Mohamed Elseidy
·Mar 27
I believe that beyond the year 2025, the difference between thriving startups and those that are falling behind comes down to one major factor, and that is, how effectively they integrate agentic pipelines into their workflows. The autonomous AI paradigm shift isn't science fiction anymore, it's here now.
We have just witnessed a breakthrough in March 2025 with the launch of Manus. The first (relatively) fully autonomous AI agent that is capable of executing diverse tasks without continuous guidance from its user. In comparison to previous AI agents, Manus can swiftly tackle various tasks simultaneously like building functional websites, analyzing data in real-time, and generating code on the fly. Its parallel and multi-agent cloud architecture represents a paradigm shift in how agentic AI operates.
The first generation of these agents won't replace humans entirely, rather, it will change how work is conducted. It won't be the organizations with the most people who will succeed, but those that can most effectively adopt these agentic-based workflows. To understand how we arrived at this inflection point, let us examine the rapid evolution from “simple” chatbots to today's autonomous digital workers.
Chatbots to Digital Workers: How did we get here?
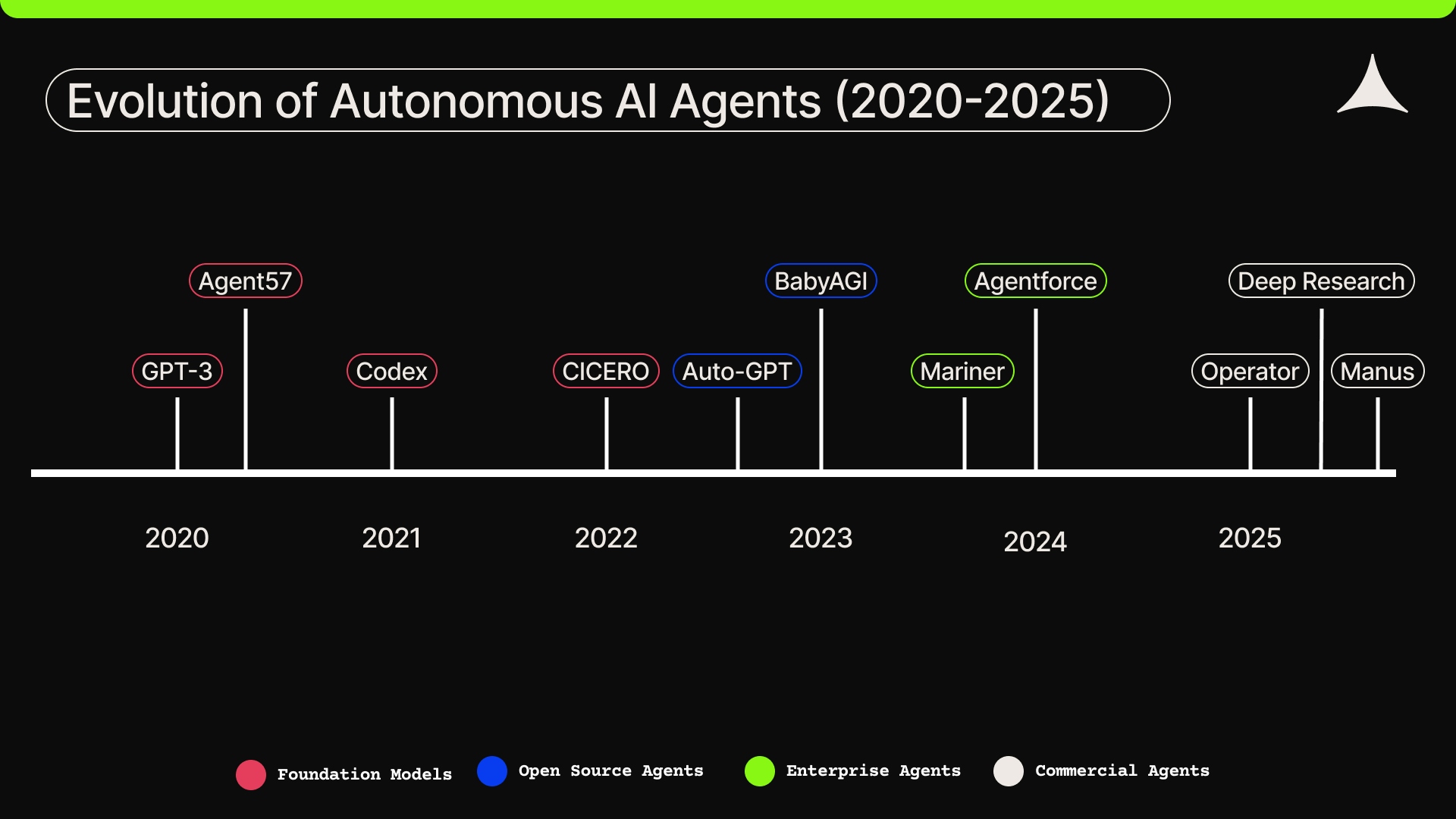
What took decades in earlier technology advancements happened in five years for AI agents. Obviously the timeline extends before that, but I will just focus on the last 5 years for now. The current timeframe from research breakthrough to product commercialization is now measured in mere months. The timeline below shows the inflection points that have shifted the AI ecosystem from "simple" text generation to taking complex actions:
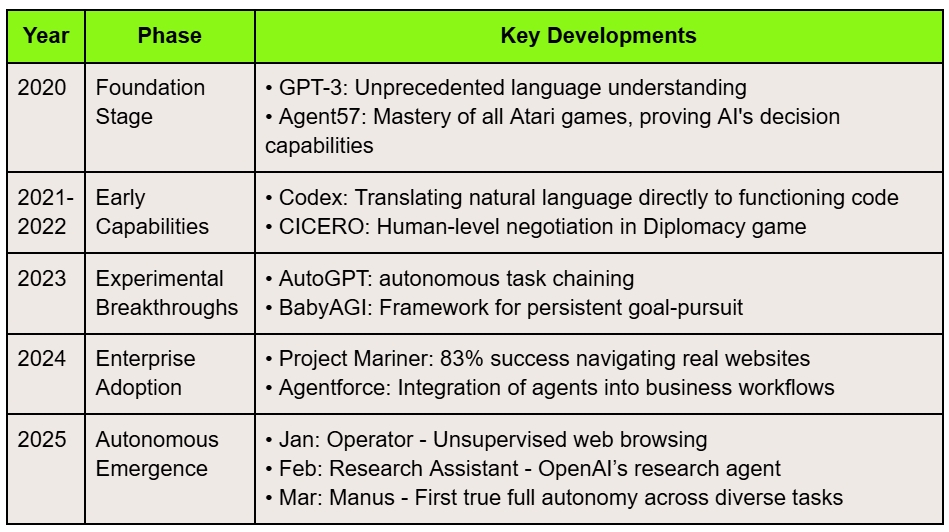
This compressed timeline illustrates the accelerating pace of innovation in the AI agent space. Notice how early 2025 began with a Cambrian explosion of commercialized agentic functionalities.
Why Manus Stands Out
To appreciate Manus's unique impact, let's examine some of the concrete applications that demonstrate its capabilities:
Development & Technical: builds complete websites in under 5 minutes and creates interactive data dashboards without manual intervention.
Financial & Analytics: Autonomously analyzes stock performance with rich visualizations of market trends
Marketing & E-commerce: Analyzes data to produce actionable recommendations on product focus and pricing. Monitors campaign performance in real-time, detecting anomalies and opportunities.
Operations & HR: Streamlines supplier sourcing with comprehensive analysis of options. Automates resume screening and interview scheduling, optimizing complex calendars with minimal conflicts
These are all applications that were developed by Manus end-to-end, with minimal human intervention. What truly separates Manus from previous AI frameworks is its innovative multi-agent infrastructure. Unlike Research Assistant's focus on information gathering or Operator's web-browsing capabilities, Manus enables task completion across diverse domains and in particular, it outperforms in the breadth of capabilities and the level of independence.
Manus also stands apart from specialized coding assistants like Claude Code, Cursor ai, or Lovable through several key factors that work together in an integrated system:
• Multi-agent infrastructure: Manus operates as a coordinated team of specialized AI agents working simultaneously, e.g., one researching, another coding, others testing, all orchestrated by a supervisor agent
• Full-cycle automation: Building on this architecture, Manus autonomously handles the entire development pipeline from designing & coding to debugging, testing, and deploying, while most AI coding tools only handle single aspects of development or require human supervision within the loop
• Asynchronous operation: This infrastructure enables Manus to work in a cloud-based environment where you can assign a complex task and return later to a completed product, rather than needing to collaborate throughout the process
• External tool integration: Manus seamlessly interfaces with various tools, like, web browsers, code editors, databases, and deployment services, enabling it to not just write code but implement and launch it.
Beyond its architecture, what makes Manus impressive is its cost-effectiveness. Reports indicate its average task cost is approximately $2, roughly one-tenth the cost of similar tasks performed by competing AI agents. This combination of autonomy and affordability makes it especially attractive for resource-conscious startups.
To better understand Manus's position in the current landscape, the following table compares key characteristics across the major AI agents available today:
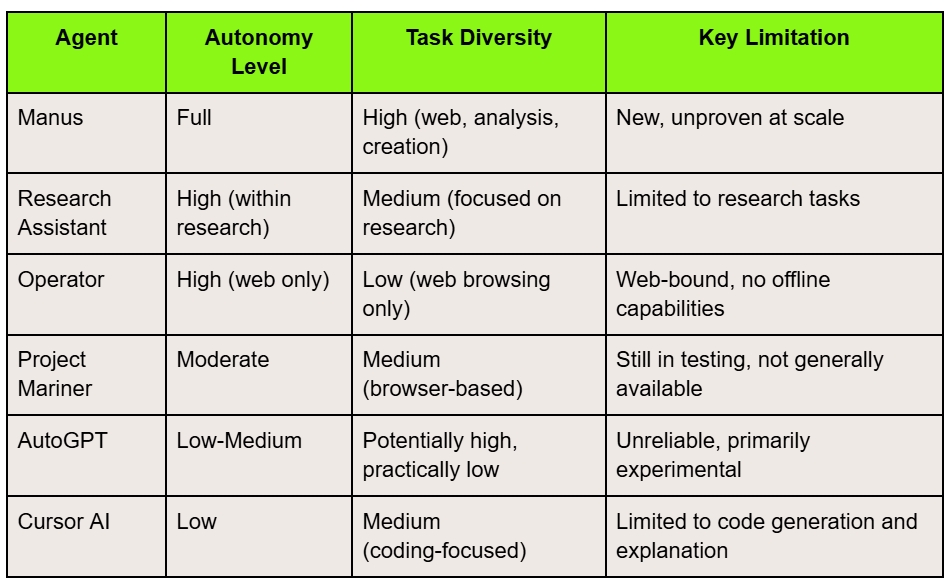
The Future Beyond Manus
Looking beyond Manus, we're likely to be entering an age where autonomous digital agents become ambient assistants rather than tools we explicitly develop. Next-generation versions will likely see deeper coordination among agents with specializations occurring behind the scenes. The upcoming breakthrough will fundamentally reorient the dynamics, focusing less on the capabilities of AI agents and more on their seamless integration into workflows without the need for explicit control. OpenAI's March 11th release of the Responses API and Agents SDK further confirms that the playing field for AI agents is evolving rapidly. Manus is by no means an endpoint but a milestone to an ongoing paradigm shift.
From Spectator to Participant: Your Action Plan
While these technologies evolve further, founders will face the critical question of how to benefit from them by integrating them into their operations. Lets delve into this, while illustrating it through an example of a B2B SaaS company scaling their product development:
Acquaintance: Start with systematically exploring the capabilities of different agentic tools like the ones mentioned in the table above. Try to identify concrete opportunities where these tools can create value for your specific pain points. For example, the product development team might explore how Research Assistant could gather information about competition and market research, while Operator could automate extracting leads from websites and forums. These tasks normally occupy hours every week that don't directly contribute to building the product
Start with Low-Risk Pilots: Once you have identified promising applications, turn this knowledge into experiments with clear success metrics. The main key point here is to choose contained projects with high potential return and minimal disruption risk. In our example, the product team might start with using Manus to analyze usage patterns for a single product line. This pilot experiments with Manus to identify user behavior patterns with comparable accuracy in comparison to manual analysis, while delivering insights in hours instead of days
Automation: After efficiency validation through pilots, try to progressively expand your implementation to cover core operational bottlenecks. This phase shifts from experimentation to integration, where agents begin handling complete workflows rather than isolated tasks. Back to our example, after some initial confidence, the product team uses Manus to further expand analytics across all modules, A/B tests, and feature recommendations. Thereby, the engineers would receive daily usage insights directly, freeing them from data analysis to focus on actual development
Measure Impact with Clear Metrics: As you scale implementation, rigorous measurement becomes essential to validate ROI and to identify optimization opportunities. Establish baseline metrics before implementation and track improvements with quantifiable data points. By measuring specific KPIs, the company in our example might document that development cycles shortened from weeks to days, feature adoption rates improved by X%, and engineering team capacity effectively increased by Y%. All this without adding headcount, simply by eliminating the data analysis bottleneck
Strategize for Industry Disruption: With proven internal success, look beyond efficiency gains toward competitive positioning. The best opportunities don’t come from doing the same things faster, but from fundamentally transforming business models around these new capabilities. In our running example, the company could shift from quarterly planning cycles to continuous data-driven development which gives them a competitive edge over those who rely on periodic reviews.
Final Thoughts
The opportunity window for first-mover advantage is closing fast. The companies that will dominate in this new era are not necessarily those with the largest resources, but those who start experimenting now with clear purpose and progression. Begin with one high-value use case today, even if small in scope, and keep evolving.
More to Read
An LLM In Your Pocket

Mohamed Elseidy
·Mar 12
With the advent of Deepseek, Qwen and others, we are witnessing a fundamental transformation in what is attainable with AI applications.
How AI Has Changed Startups Forever
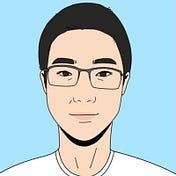
Qiao Wang
·Feb 20
When ChatGPT first came out three years ago, I wrote a wrapper to help myself prioritize emails and messages. My coding skills were rusty, so I used ChatGPT itself as a coding copilot. I instantly felt the magic. It took me maybe half a day to finish the project, while it would normally take me a few days in the pre-AI era.
2024 Crypto Startup Trends Report
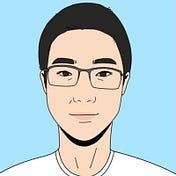
Qiao Wang
·Oct 21, 2024
In this report, we provide trends observed in our application data and insights as to what they indicate for the broader startup ecosystem.